28 Jun 2023
This is a follow-up to my recent post trying to implement the classic
Conway Game of Life in an MVU style with Avalonia.FuncUI. While I managed
to get a version going pretty easily, the performance was not great. The
visualization ran OK until around 100 x 100 cells, but started to degrade
severely beyond that.
After a bit of work, I am pleased to present an updated version, which runs
through a 200 x 200 cells visualization pretty smoothly:
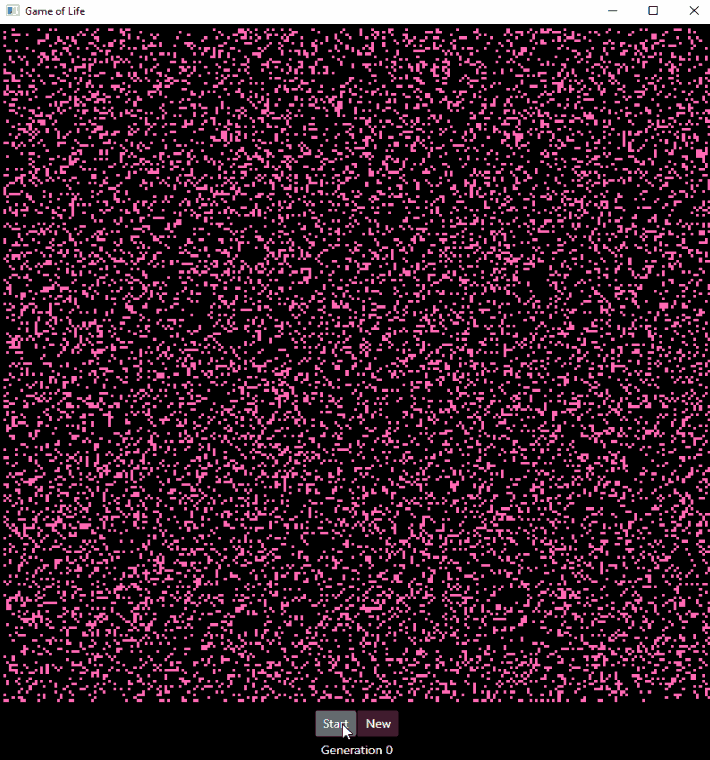
As a side note, I wanted to point out that the size change is significative.
Increasing the grid size from 100 to 200 means that for every frame, the
number of elements we need to refresh grows from 10,000 to 40,000.
In this post, I will go over what changed between the two versions.
You can find the full code here on GitHub
More...
17 Jun 2023
A couple of days ago, I came across a toot from Khalid Abuhakmeh,
showcasing a C# + MVVM implementation of the Game of Life on Avalonia. I
have been experimenting with Avalonia funcUI recently, and thought a conversion
would be both a fun week-end exercise, and an interesting way to take a look at
performance.
Long story short, I took a look at his repository as a starting point, and
proceeded to rewrite it in an Elmish style, shamelessly lifting the core from
his code. The good news is, it did not take a lot of time to get it running,
the less good news is, my version has clear performance issues.
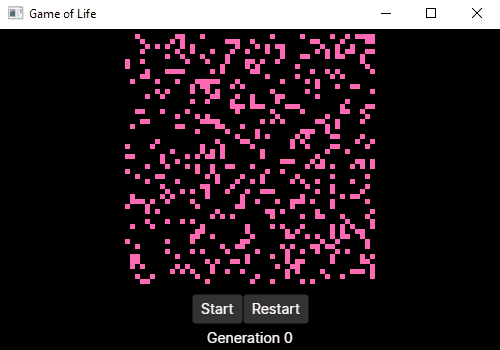
In this post, I will go over how I approached it so far, and where I think
the performance issues might be coming from. In a later post, I’ll try to see
if I can fix these. As the French saying goes, “A chaque jour suffit sa peine”.
You can find the full code here on GitHub
More...
29 May 2023
In the recent weeks, I came across a use case which sounded like a good fit for
a desktop application, which got me curious about the state of affairs for .NET
desktop clients these days. And, as I was looking into this, I quickly came
across Avalonia, and specifically Avalonia.FuncUI. Cross platform
XAML apps, using F# and the Elmish loop? My curiosity was piqued, and I figured
it was worth giving it a try.
In this post, I will go over my first steps trying the library out. My
ambitions are limited: first, how hard is it to get something running? Then,
how hard is it to take an existing Avalonia library (in this case, the
charting library OxyPlot), and bolt it into an Elmish style Avalonia app?
You can find the full code here on GitHub
More...
15 Apr 2023
Some time back, I wrote a small post digging into the
mechanics behind the Nelder Mead solver. As it turns out, I had a use for
it recently, and after copy-pasting my own code a few times, I figured it would
make my life easier to turn that into a NuGet package, Quipu.
So what does it do, and why might you care?
A code example might be the quickest explanation here. Suppose that, for
whatever reason, you were interested in the function f(x) = x ^ 2
, and wanted
to know for what value of x
this function reaches its minimum.
That is easy to solve with the Quipu Nelder-Mead solver:
open Quipu
open Quipu.NelderMead
let f x = x ** 2.0
let solution =
NelderMead.solve
Configuration.defaultValue
(Objective.from f) [ 100.0 ]
printfn $"{solution}"
… which produces the following result:
Optimal (0.0001556843433, [|0.01247735322|])
The function f
reaches a minimum of 0.0001
, for x = 0.0124
.
More...
08 Jan 2023
This post is intended primarily as a note to myself, keeping track as my findings
as I dig into automatic differentiation with DiffSharp. Warning: as a result,
I won’t make a particular effort at pedagogy – hopefully you’ll still find something
of interest in here!
The main question I am interested in here is, how can I use DiffSharp to find the
minimum of a function? I will take a look first at basic gradient descent, to get us
warmed up. In a future installment I plan to explore using the built-in SGD and Adam
optimizers for that same task.
The full code is here on GitHub, available as a .NET interactive notebook.
Test function
The function we will be using in our exploration is the following:
$f(x,y)=0.26 \times (x^2+y^2) - 0.48 \times (x \times y)$
This function, which I lifted this function from this blog post,
translates into this F# code:
let f (x: float, y: float) =
0.26 * (pown x 2 + pown y 2) - 0.48 * x * y
Graphically, this is how the function looks like:
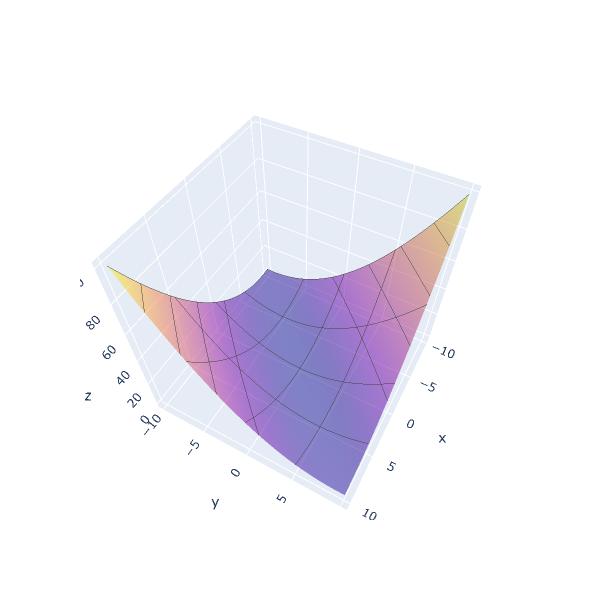
This function has a global minimum for (x = 0.0, y = 0.0)
, and is
unimodal, that is, it has a single peak (or valley in this case). This
makes it a good test candidate for function minimization using gradient
descent.
More...